Amari is ADGA’s next-generation machine learning platform for deriving actionable intelligence from earth observation datasets. It provides a specialist framework to rapidly train neural networks without human intervention, allowing observers not just to keep pace with the already overwhelming and always increasing volume of available satellite imagery, but to bring observation and detection as close to real time as possible while doing so.
With hundreds of new satellites scheduled for launch in the next few years, the volume of input data will only intensify. Amari steps in where traditional statistical approaches simply cannot keep up. Building on our proven expertise in computing for space and satellite applications, Amari provides context-specific training and detection abilities for a wide array of uses, from national security to academic research.
Key potential applications include:
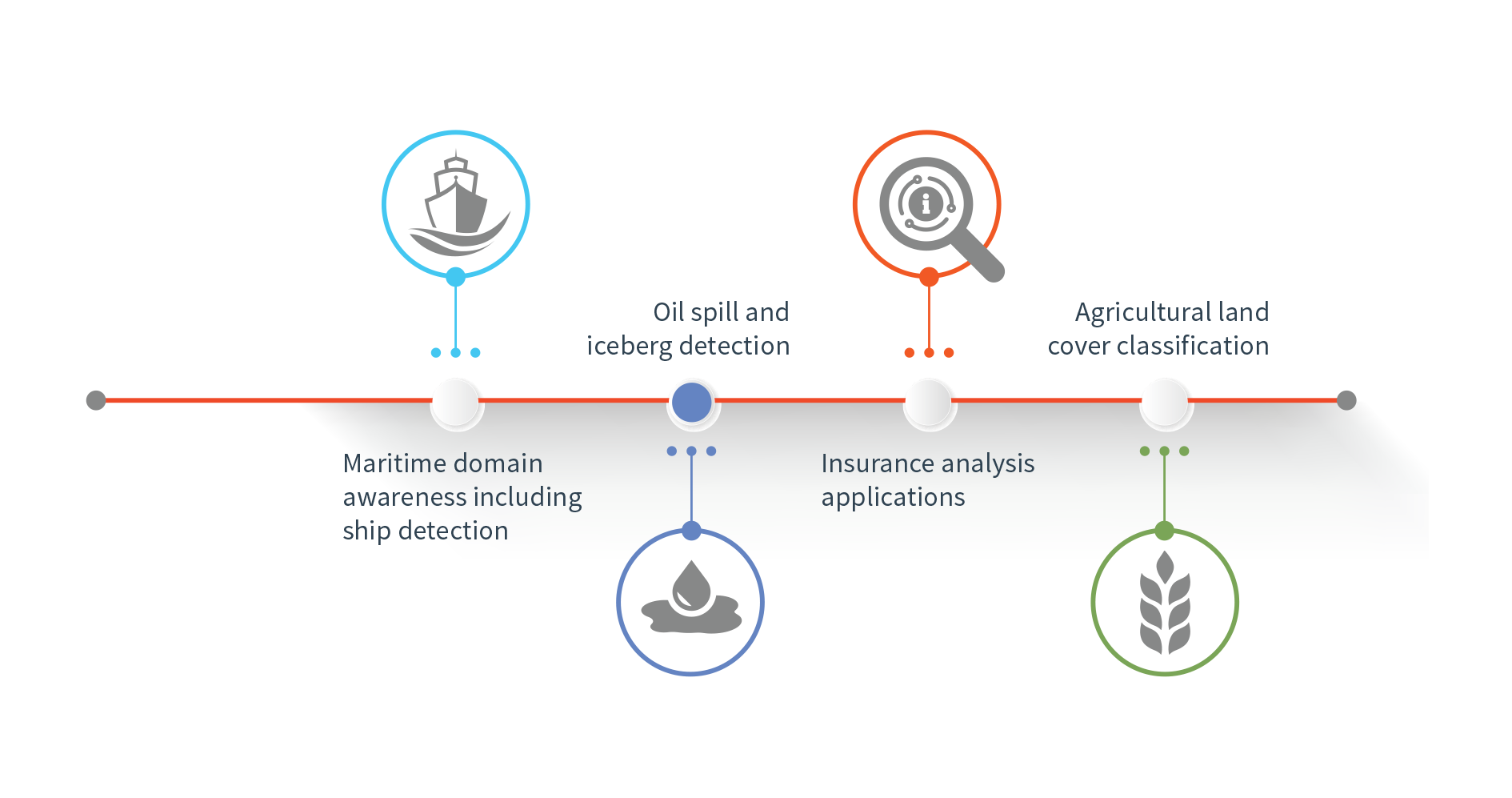
Training Fast Through Transfer Learning
Built on an entirely open-source technology stack, with Google’s TensorFlow framework at its core, Amari can operate in a private or hybrid cloud environment, depending on security requirements.
Amari can operate and adapt at pace by repurposing existing image recognition learning models, trained for detecting everyday objects, in a manner suitable for both radar and optical satellite imagery. Neural networks can be trained on specialist data sets from this baseline through a process known as transfer learning, leveraging pre-existing and no cost generic learning models towards highly specialized outputs using a relatively small amount of new training data. It does not require manually coded rules and can be trained at speed for a limitless variety of identification tasks. As computational costs are largely incurred at the time of initial training, Amari’s small in-operation footprint allows for deployment in edge environments, near to data origin or at ground stations.
Amari will soon be available for satellite imaging providers, data brokers, and other organizations with data needs as a managed service or to be used in house. ADGA also offers licensing of Amari training data and output data sets for use in research and other applications.
Amari at Work: A New Frontier in Maritime Domain Awareness
At any given time, there are more than 100,000 ships in the world’s waters. A wide variety of observers, from defence organizations to environmental monitors, require information as to where they are in near real time. Ship detection approaches based on self-reporting, such as via transponder, can be susceptible to manipulation through spoofing, or by dark targets who have disabled their equipment. Active surveillance imaging technologies like Synthetic Aperture RADAR allow for generation of high-trust, all weather, day and night monitoring data, but existing computerized analysis techniques based on statistical evaluation still require human intervention for certainty, and simply cannot maintain quality at the speed required to process all available incoming data.
Amari’s ship detection neural network, trained on imagery from the European Space Agency’s Sentinel-1 and Canada’s RADARSAT Constellation Mission (RCM), detects and reports vessel signatures in a lightweight and rapid manner. Expansion for more complete maritime domain awareness—monitoring icebergs, ship wakes, and oil spills—requires only modifications to the training dataset, not a change in the underlying tools or code. Amari’s trained models improve and adapt through use as the datasets grow over time.
Aspects of Amari’s development have been supported by funding through the Canadian Space Agency, as ADGA prepares to trial the platform with a range of stakeholders for global implementation in a variety of maritime domain awareness contexts.